In today’s fast-changing digital world, how we process and analyze data has changed a lot. As someone who works in this field, I’ve seen big improvements in artificial intelligence (AI) and data use. Now, I want to take you on a journey to see how what is cloud computing and edge AI work together.
Edge AI’s rise shows its huge potential. Combining Edge and Cloud AI can give companies the best of both, improving operations, security, and innovation while keeping data safe.
Edge AI brings processing power to devices, making things faster. Cloud AI uses lots of resources for complex AI models. The choice depends on data volume, goals, and other factors.
In short, picking between edge AI and cloud computing is complex. It’s about finding the right balance of speed, security, and cost. By knowing the strengths and weaknesses of each, businesses can create a strong AI system that fits their needs.
Choosing between cloud computing and edge AI depends on your application’s needs. Understanding each technology’s strengths and weaknesses helps you make the best choice for your situation.
Understanding Edge AI and Cloud Computing
Technology keeps getting better, leading to two main ways to use artificial intelligence (AI): Edge AI and Cloud Computing. Each has its own benefits and things to think about. It’s important to know how they differ.What is Edge AI?
Edge AI means running AI on devices like IoT sensors and edge servers. This way, data is processed right away, without sending it to a cloud. The edge AI market is growing fast, expected to hit over $107 billion by 2030. AI devices are becoming more common, with 29 billion expected by 2030. This growth is driving the edge AI market.What is Cloud Computing?
Cloud computing uses remote servers for computing, storage, and software over the internet. Companies like Google Cloud, AWS, and Microsoft Azure offer cloud AI services. They let users use the cloud’s power for their AI needs.Key Differences in Architecture and Processing
Edge AI and cloud computing differ in how they work. Edge AI processes data where it’s collected, making decisions quickly. This cuts down on latency and boosts efficiency. Cloud computing, on the other hand, uses a big, central system. It offers lots of power and storage but has more latency because of data transmission. Cloud computing has more power and can grow bigger. But edge AI has its own perks, like working offline and keeping data private. Edge AI also saves money by cutting down on cloud costs.The Cloud Computing Paradigm
Cloud computing is key for AI growth, offering big advantages like scalability and flexibility. It gives access to powerful computing and centralizes data processing. Big names like AWS and Google Cloud offer tools for better data analysis. It’s perfect for tasks needing lots of power and storage, helping teams and startups.Advantages of Cloud-Based AI
Cloud computing brings many benefits to AI:- Scalability: Clouds can grow to meet AI needs.
- Flexibility: AI solutions can quickly change to fit business needs.
- Accessibility: Clouds make top AI tools available to all, making AI development open to more people.
- Centralized data processing: Clouds handle big data for AI training and use well.
Limitations of Cloud-Based AI
But, cloud-based AI also has downsides:- Latency issues: Clouds can slow down, hurting apps that need fast responses.
- Connectivity requirements: Cloud AI needs stable internet, failing without it.
- Data privacy and security concerns: Clouds raise worries about keeping data safe and private.
- Ongoing operational costs: Cloud fees add up, affecting business budgets.
The Rise of Edge AI
Edge AI is changing the tech world by making data processing faster and more immediate. It solves cloud computing’s problems by handling data on devices. This brings many advantages of Edge AI, like quick processing, less delay, better privacy, and less data use.Advantages of Edge AI
- Real-time processing: Edge AI devices quickly analyze and act on data, making decisions fast.
- Lower latency: It cuts down on delay by processing data locally, making apps more responsive.
- Enhanced data privacy and security: Keeping data on devices reduces breach risks and meets privacy rules.
- Reduced bandwidth usage: Processing data locally means less need for cloud data transfer, saving network resources.
Limitations of Edge AI
Edge AI has many benefits but also some limitations. These include limited computing power, higher costs for hardware, and harder system updates.- Computational constraints: Edge devices have less memory and power than clouds, limiting AI model complexity.
- Higher initial hardware investment: Setting up Edge AI needs special hardware, raising costs for companies.
- Challenges in maintenance and updates: Updating Edge AI across many devices is complex, needing more resources and coordination.
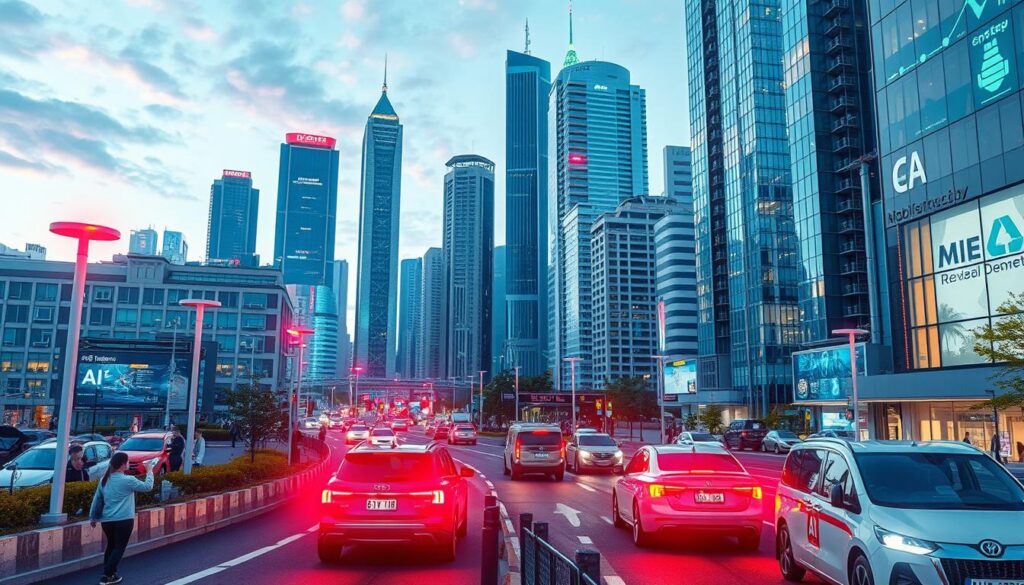
What is Cloud Computing and Edge AI
Cloud AI | Edge AI |
---|---|
Scalability and large-scale data processing capabilities | Reduced latency and enhanced privacy by keeping data local |
Relies on strong internet connectivity | Limited by computational and storage constraints of edge devices |
Faces latency issues | Challenges in maintaining and updating distributed systems |
Edge vs. Cloud
The debate between edge AI and cloud computing is heating up. Businesses are trying to pick the best tech for their needs. Cloud computing offers lots of power and storage, but edge AI is faster and more secure for real-time tasks. Cloud computing uses big data centers far away. On the other hand, edge computing is everywhere, near devices. This makes a big difference in how fast things work and how much it costs. Cloud computing is good for tasks that don’t need to be done quickly. But edge computing is perfect for tasks that need to happen fast.Feature | Edge Computing | Cloud Computing |
---|---|---|
Latency | Low latency, real-time processing | Higher latency, not suitable for time-sensitive applications |
Connectivity | Can operate without internet connectivity | Requires internet connectivity |
Cost | Automated scalability, zero-touch provisioning | Expensive and resource-intensive operational activity |
Security and Privacy | Reduced attack vectors, enhanced privacy | Higher security risks due to centralized data storage |
What is Cloud Computing and Edge AI
In today’s digital world, cloud computing and edge AI are changing the game. Knowing the difference between these two is key to finding the right solution for you. What is Cloud Computing? Cloud computing uses remote servers to offer computing power, storage, and software over the internet. It lets users access and manage data and apps from anywhere, without needing local hardware. What is Edge AI? Edge AI runs AI algorithms directly on devices like IoT sensors and edge servers. This approach brings computing power closer to data sources. It enables fast processing and decision-making, avoiding the delays of cloud-based AI. Cloud computing and edge AI differ in their setup and how they process data. Cloud computing uses a central infrastructure, while edge AI spreads out data analysis across devices. This edge advantage can lead to quicker responses, better privacy, and less bandwidth use.Cloud Computing | Edge AI |
---|---|
Centralized infrastructure with remote servers and data centers | Distributed network of devices at the edge of the network |
Processes data in remote data centers | Processes data at the source, close to the devices |
Requires a stable internet connection for data transfer | Can operate with or without an internet connection |
Scalable computing power and storage | Limited computational resources on edge devices |
The Cloud Computing Paradigm Continued
The cloud computing model has big advantages for businesses. It lets companies easily grow or shrink their computing needs without big upfront costs. This model also makes it easy to access powerful computing and data processing over the internet. But, there are also limitations. Latency issues can happen when data moves from devices to the cloud. The need for reliable internet to use cloud services is another challenge. Also, keeping data safe and private is a big concern since it’s stored on remote servers. These challenges have led to the growth of edge AI. Edge AI tries to solve cloud computing’s problems by processing data locally. This reduces delays and helps with internet and security issues.Cloud Computing Advantages | Cloud Computing Limitations |
---|---|
|
|
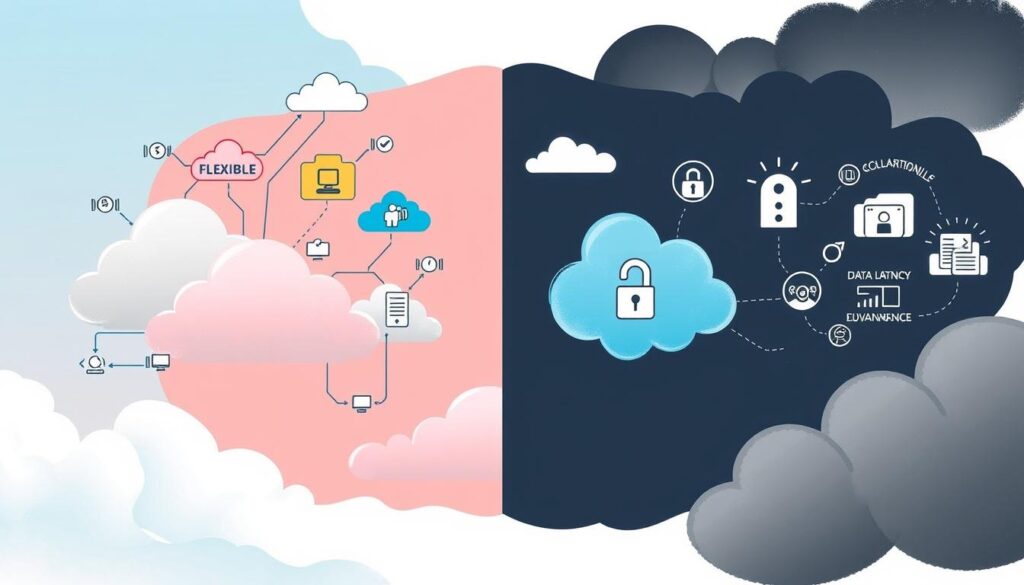
What is Cloud Computing and Edge AI
“Edge computing brings the power of cloud computing closer to customer premises at the network edge to compute, analyze, and make decisions in real time.”
Edge AI Applications and Use Cases
Edge AI is changing many industries as edge computing grows. It offers real-time processing and decision-making. This is making big changes in different areas and bringing real benefits. In industrial IoT, edge AI makes monitoring and response faster. For example, it can watch over equipment, spot problems, and fix them quickly. This makes operations more efficient and safer. Healthcare is also seeing big changes with edge AI. Medical devices and systems with edge AI help care for patients right away. They help make quick decisions and improve health outcomes. Edge AI also helps protect finances by spotting unusual spending. These examples show how edge AI is valuable where speed and reliability matter. It processes data locally, cutting down on cloud communication. This saves bandwidth and boosts system performance. Edge AI is used in many areas, like airport security and quality control in factories. As we connect more things through the Internet of Things (IoT), edge AI will be key. It will help bring new and important solutions to many fields.“Edge AI models can be deployed locally, eliminating the need for sending API requests or real-time data to cloud servers for inference.”
Integrating Edge and Cloud AI
Combining edge AI and cloud AI creates a powerful AI system. It uses the best of both worlds. This mix balances edge AI’s quick processing with cloud AI’s deep analysis and big computing power. This combo boosts model performance. Edge AI makes fast changes based on current data. Cloud AI refines models over time with more data.Benefits of a Hybrid Approach
Using edge ai and cloud ai together has many benefits:- Less delay: Edge AI works locally, giving fast responses. This is key for self-driving cars and health checks.
- Cost savings: The edge cloud ai hybrid cuts down on data sending costs. Only key data goes to the cloud for deeper checks.
- Better security and rules: Handling data locally boosts security and follows rules better.
- More reliability: The edge cloud ai hybrid keeps working even when internet is spotty. This lowers risks.
Challenges and Considerations
While the benefits of edge cloud ai hybrid are great, there are also challenges of edge cloud ai hybrid to face:- Complex design: Merging edge and cloud needs careful planning and matching.
- Keeping data in sync: It’s key to have smooth data flow and sync between edge and cloud for a unified AI model.
- Security and rules: Protecting the whole edge ai and cloud ai integration setup and following data rules are vital.
- Special skills needed: Running a hybrid edge cloud ai system requires specific knowledge and skills.
Conclusion
Cloud computing and edge AI are changing how we process and analyze data. Cloud computing gives us lots of computing power and stores data in one place. On the other hand, edge AI makes decisions quickly, right where the data is. Businesses need to think about what each technology offers. They should decide if they want to use cloud, edge, or a mix of both. This choice depends on their specific needs. The need for quick, local data processing is growing. Edge AI and cloud AI will be key in this new era. The key takeaways cloud computing and edge ai show we need a balanced approach. This way, we can meet the changing needs of the digital world. By understanding the conclusion cloud computing and edge ai, businesses can make smart choices. They can pick the right mix of cloud and edge AI to reach their goals. The future of data processing will be shaped by cloud computing and edge AI. This will help organizations make quicker, smarter decisions. It will also keep data safe and private. As technology keeps changing, working together will be crucial. Cloud and edge AI will unlock new possibilities. They will drive big changes across different industries.FAQ
What is the difference between edge AI and cloud computing?
Edge AI runs AI algorithms on local devices, allowing for quick data processing. Cloud computing uses remote servers for computing power and storage over the internet.
What are the advantages of edge AI?
Edge AI is great for real-time processing and low latency. It also keeps data private and uses less bandwidth.
What are the limitations of edge AI?
Edge AI has limits like processing power and high initial costs. It’s also hard to keep systems updated.
What are the advantages of cloud computing for AI?
Cloud computing is scalable and flexible. It gives access to powerful resources and centralizes data processing.
What are the limitations of cloud-based AI?
Cloud AI has latency and connectivity issues. It also raises data privacy and security concerns, and costs money to run.
How can edge AI and cloud computing be integrated?
Combining edge AI and cloud AI creates a strong AI system. It uses edge for quick processing and cloud for deep analytics. But, it’s complex to set up and requires system compatibility.
What are some real-world applications of edge AI?
Edge AI is used in many fields. It helps with immediate monitoring in industrial IoT and real-time patient care in healthcare.